Research Funding
- NSERC Alliance and Mitacs joint program, "Residential Electrification: Power Grid Impact, Mitigation Strategies and Environmental Benefits", 2024-2027, PI.
- NSERC Alliance, "Electric vehicle infrastructure design, optimization, and emission evaluations toward full- scale net-zero transportation sector", 2023-2027, Co-PI
- NSERC Alliance preparatory funds, "Sustainable, Profitable, and Net-zero Controlled Environment Agriculture," 2023, PI.
- NSERC Catalyst, "Design, modeling and optimization of a hybrid wind-gravity energy system," 2023-2024, PI.
- Mitacs Accelerate Grant, "Clean Heating Using Geothermal Heat Pump Technology: A Feasibility Study For The City Of Regina," 2023-2025, Co-PI.
- Mitacs Accelerate Grant, "Sustainable Geothermal Reservoir Energy Production, Conversion, Utilization and Energy System Integration by Using Carbon Dioxide," 2023-2027, Co-PI.
- Mitacs Accelerate Grant, "Design and development of a Virtual Window with industrial and civil applications," 2023-2024 (PI)
- Mitacs Accelerate Grant, "Sustainable Geothermal Reservoir Energy Production, Conversion, Utilization and Energy System Integration in Saskatchewan Province," 2023-2025 (Co-PI)
- NSERC Discovery Grant, "Integration of distributed energy resources into electricity systems and markets," 2021-2026 (PI)
- Mitacs Accelerate Grant, "Integration of electric vehicles into the power distribution system," 2021-2023 (PI)
- Mitacs Research Training Award, "Multi-agent optimization for vehicle-to-grid applications in frequency regulation," 2020 (PI)
- Mitacs Research Training Award, "Vehicle to Grid Application in Unit Commitment," 2020 (PI)
- President's Research Seed Grant at the University of Regina, "Stochastic Optimization for Residential Demand Response in Power Systems and Electricity Markets," 2020-2022 (PI)
- Research Opportunities Fund at the University of Regina, "Demand Response for Solar PV Energy Integration," 2021 (PI)
- Research Start-Up Fund at the University of Regina (PI)
Sponsors and Partners (in alphabetical order)
I would like to express my sincere gratitude to our sponsors and partners. Without their generous support, the research in our group would never be possible.
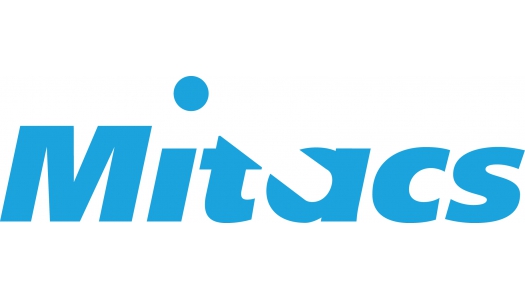
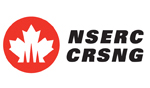
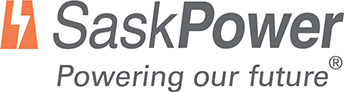
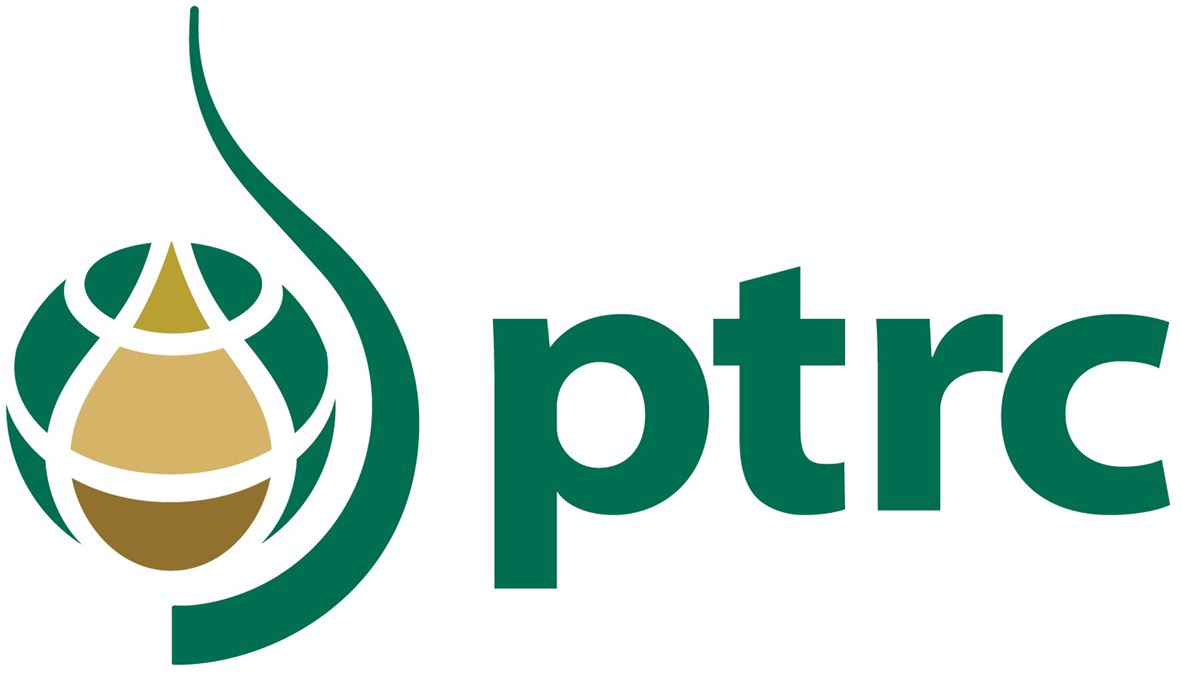
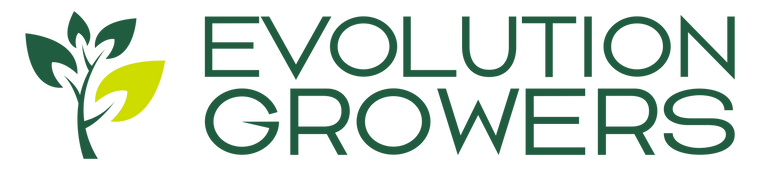